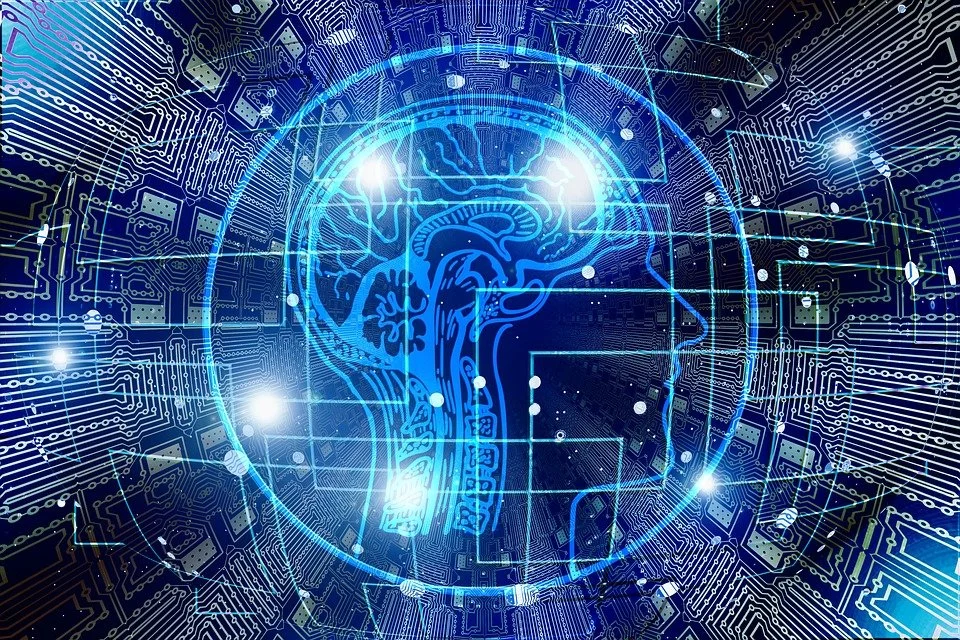
Theme 3: Data Driven Innovation
UK Digital Steel Innovation Hub (DSIH)
Intelligent Steel Production
To be transformative, the industry will have to be disruptive. The goal of this task is to ultimately generate disruptive technologies for 21st Century supply chains and business models.
New approaches to process modelling and optimised fast-algorithm techniques will be used to allow real-time simulation and prediction of complex thermodynamic, kinetic and mechanical processes.
Task 4: Digital Steel Innovation Hub (DSIH)
Project Leaders: Professor Janet Godsell, Professor Giovanni Montanna, Professor Arnold Beckmann, Professor Cinzia Giannetti
Researchers: David Ireland, Dr Kayal Lakshmanan
Project Partners: WMG University of Warwick, Swansea University, British Steel, Celsa Steel UK, Sheffield Forgemasters, Liberty Steel, Tata Steel UK, nChain
Introduction
T4 aims to create a data-driven step-change improvement in the competitiveness of UK steel supply chains (SCs). It will utilise recent developments in technologies to both harness and analyse data that can improve the value-added and productivity of products, processes, and UK steel industry SC. The project will explore how the adoption of industrial digital technologies (IDTs) could improve steel SC productivity and sustainability. Deep reinforcement learning algorithms will be developed to train multiple robotic agents to collaboratively solve tasks such as object manipulation using direct input from mounted cameras. In addition, the project will assess the use of a demonstrator tool in enabling real-time predictive maintenance and facilitating product-related knowledge and data sharing among different stakeholders.
Planned impact
To improve competitiveness of UK steel supply chains in a sustainable and value-adding way
Progress to date
Digital Supply Chain
Conducted SC Digital Readiness survey to industrial partners
Conducted a workshop with the industrial partners to share SC Digital Readiness results
Developed an Industrial Digital Technologies (IDTs) guide
Conducted a semi-structured interview with an industrial partner to verify the IDTs guide
Conducted a case study with Tata Steel and Moveero to optimise SC productivity, sustainability and carbon emission
Semantic Technologies
Common Reference Ontology for Steelmaking (CROS)
Conceptualization of Stream Reasoner to continuously query and reasoning on real-time data
Hybrid Modelling
Combining physics-based steady-state model and data-driven deep learning model to improve the prediction efficiency of Silicon in Blast Furnace (T4, T5).
Raw Si vs predicted Si w.r.t number of time samples using CNN+LSTM deep learning model. R2 score, MS and MAE is 0.75, 0.08, and 0.06.
Company case studies
January 2020: Organised a workshop with all industrial partners and agreed to have a 2-stage case study approach:
Stage 1 - Liberty Steel and Forgemasters
Stage 2 - Tata Steel and British Steel
Due to COVID-19, project partners were severely affected with a large number of key staff furloughed. As a contingency, we adapted our approach by re-aligning our existing SC research projects. A joint project between SUSTAIN, Transforming Construction and ESRC Productivity was formed to explore the concept of SC productivity across different sectors. As a result, a large-scale survey was developed. The research output will be made available in the form of industry reports and a peer-reviewed academic journal paper.
October 2020: Re-engaged with Liberty Steel as furlough reduced. The project has been re-scoping and the focus shifted to steel demand from aerospace customers (e.g. Boeing) to the Bolton Air Service Centre and then to the Stocksbridge site. Detailed research design was developed and the approach could be adopted by other steel companies.
Key findings
Results from the SC Digital Readiness (SCDR) assessment show that steelmakers have a lower digital maturity level
Initial case study results indicate six sources of misalignment gaps within and between organisations
Key Publications
Task 5: Intelligent Steelmaking
Project Leaders: Dr Richard Thackray, Dr Michael Auinger
Researchers:
Project Partners: University of Sheffield, WMG University of Warwick
Introduction
Many existing process models for steel production do not allow for process alignment and are too complex for meaningful real-time predictive use. The primary aim of Task 5 is to take a different view on steel production in its entirety by not seeking to improve product qualities but by focussing on decreasing energy usage and building links over the entire process chain. This will be achieved by development and optimisation of process level models supported by experimental verification, analysis of process data and by benchmarking current process routes to quantitatively assess how efficiently industry currently uses both energy and materials.
Project impact
Process models to optimise for energy efficiency, cost, reducing waste, compositions
Real-time decision making
An integrated and transparent picture of internal resource flows
Greater reuse of by-products and reduce energy use
Industry Context
The context to industry is to make use of existing (old) programmes and develop new fast modelling routines for fast predictions. Depending on the interest of the industrial partner, this can be relevant for optimising day-to-day process strategies or develop mitigation scenarios for unwanted production stops or equipment breakdown.
Progress to date
Sheffield:
Collection of data including material and energy input and material output for 2 electric arc furnaces and 3 ladle furnaces for 365 days
Information specifically relating to rebar production to allow for an LCI/LCA to be performed
Warwick:
Developed an adaptive process modelling approach for transport, combining physical modelling and machine learning elements. Model serves as a template for further processes in the “digital refinery”
Schematic reaction zone model for a blast furnace
Key findings
Process models which learn directly from process data can outperform parameterbased
models
Initial results from analysis of the Liberty Steel data shows that LCI/LCA and energy flows are a suitable way to measure and quantify material and process efficiency
Roadmap to impact
Working with Tata Steel UK, in order to model blast furnace stoves to allow us to create models that perform well in imperfect conditions. Well performing models will reduce costs, and increase energy efficiency.
Completion of LCA/material efficiency work which will allow Liberty to identify areas of current inefficiencies, and provide quantifiable benefits.